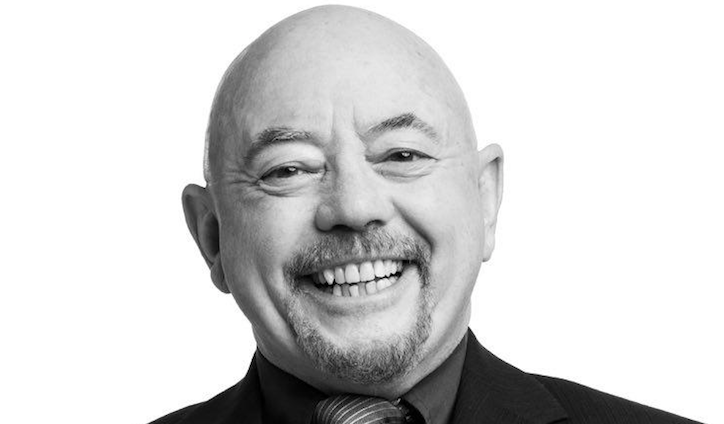
Lead Instructor
John R. Williams is a Professor of Information Engineering and Civil and Environmental Engineering at MIT. His area of specialty is large scale computer analysis applied to both physical systems and to information.
John R. Williams is a Professor of Information Engineering and Civil and Environmental Engineering at MIT. His area of specialty is large scale computer analysis applied to both physical systems and to information.
Stefanie Jegelka is an X-Consortium Career Development Associate Professor in the Department of Electrical Engineering and Computer Science at MIT, where she is a member of CSAIL, and affiliated with IDSS.
Tommi Jaakkola is a Thomas Siebel Professor of Electrical Engineering and Computer Science and the Institute for Data, Systems, and Society. He is also a member of the Computer Science and Artificial Intelligence Laboratory. His work pertains to inferential, algorithmic and estimation questions in machine learning, including large scale probabilistic distributed inference, deep learning, and causal inference.
Regina Barzilay is a School of Engineering Distinguished Professor for AI and Health in the Department of Electrical Engineering and Computer Science and a member of the Computer Science and Artificial Intelligence Laboratory at the Massachusetts Institute of Technology.
Dr. Abel Sanchez is the Executive Director of MIT's Geospatial Data Center, pioneering advancements in IoT, Big Data, AI, and Cybersecurity. He architected "The Internet of Things" global network and data analytics platforms for major corporations like SAP, Ford, Johnson & Johnson, Accenture, Shell, Exxon Mobil, and Boeing. In cybersecurity, Dr. Sanchez designed Cyber Ranges for the Department of Defense and a password firewall for IARPA. His machine learning work includes developing fraud detection frameworks for Accenture. Over the past three years, he has focused on Generative AI, building assistants, agents, and intelligence for sectors such as retail, finance, and government. Dr. Sanchez has also led e-Education software for Microsoft and co-founded the Accenture Technology Academy. He teaches MIT courses on cybersecurity, engineering computation, and data science, has produced over 1000 educational videos, and has taught professionals in 106 countries. He is a distinguished faculty member at MIT Professional Education, specializing in digital transformation.
Vivienne Sze is an associate professor in MIT’s Department of Electrical Engineering and Computer Science and leads the Research Lab of Electronics’ Energy-Efficient Multimedia Systems research group. Her group works on computing systems that enable energy-efficient machine learning, computer vision, and video compression/processing for a wide range of applications, including autonomous navigation, digital health, and the internet of things.