Why AI Can’t Fix a Vague Survey Strategy
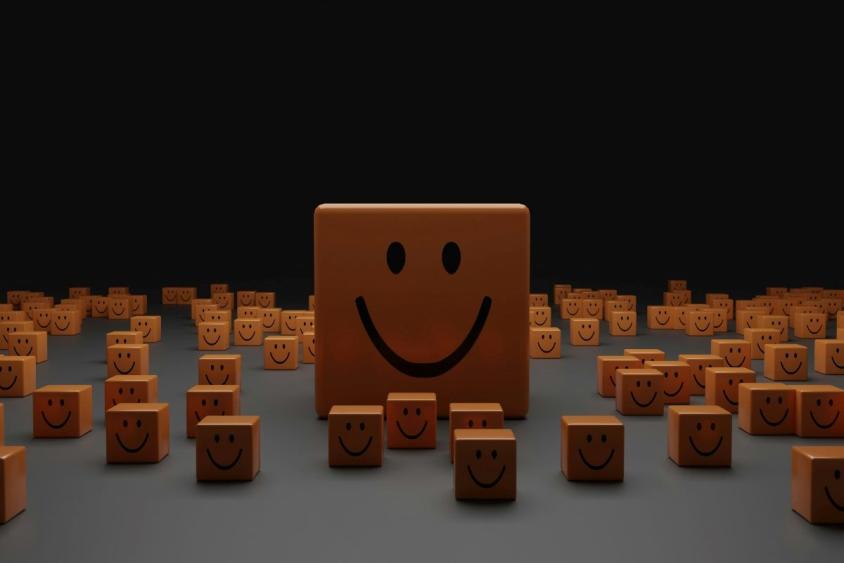
The Gist
Better in means better out. Strong inputs like trusted questions and reference material help LLMs produce more accurate, usable customer survey drafts.
Context shapes results. Vague prompts confuse AI the same way they confuse people. Narrow questions lead to sharper, more relevant outputs.
AI is a partner. Treating generative tools like collaborators rather than shortcuts leads to more useful, human-centered work.
Prompting a generative AI tool like ChatGPT is a lot like asking a good survey question. In both cases, you’re essentially having a conversation. But it's a highly structured conversation where you’re hoping to get a certain type of output. And, just like in customer survey design, the way you ask ChatGPT a question will typically have an enormous impact on the answer you get.
Strategic use of large language models (LLMs) can help survey designers speed up and even improve their work. But if researchers don’t take the time to learn the best ways to prompt these tools, they’re going to get subpar results.
Like everyone else, I’m still learning how to optimize my use of LLMs. (And the tools themselves are evolving every day, it seems.) But here are the lessons I’ve learned so far about using them to design better surveys.
Table of Contents
- Start With Better Input for Your Customer Surveys
- Focus Your Prompts for Your Customer Surveys
- Define the Destination
- Make It a Conversation
- Use AI Where It Helps
- Have AI Grade Its Own Work
- Summary of Key Lessons: Designing Better Surveys With Generative AI
Start With Better Input for Your Customer Surveys
Recently, I needed to summarize one of my talks in a blog post, and I leaned on Claude (the Anthropic LLM that many prefer for writing-related tasks) to generate a first draft. But instead of starting from scratch, I fed the model my slides, some relevant chapters from my book and even some blog posts on unrelated topics to make sure Claude could mimic my voice and style. Now, I didn’t submit Claude’s draft as a final copy, but it served as a helpful jumping-off point for my own draft.
The same can be true for surveys. But, just as with a blog post, you shouldn’t go into the process cold. Instead, feed the LLM materials like survey questions from a reputable source like Pew Research Center. The better your inputs are, the better your outputs will be.
Focus Your Prompts for Your Customer Surveys
This is an area where survey design and AI prompting mimic one another. If you ask a survey respondent a vaguely worded question like, “How are you doing today?” that leaves a lot of room for misinterpretation.
The same is true when prompting an LLM like ChatGPT or Claude. These tools are designed to provide you an answer even if they lack the necessary context or information (a phenomenon popularly called “hallucination”), and they will fill in any gaps with unhelpful fluff. Instead of telling an LLM to write survey questions about trust in healthcare, instruct the model to design a question about people’s trust in their primary care doctors, using a seven-point Likert scale.
Related Article: Top 5 Generative AI Prompt Tips for CX and Marketing Leaders
Define the Destination
Too often, people show up to an LLM with only a vague idea of what they’re looking for, trust the AI model to do their work for them and then walk away disappointed with the end product. But if you don’t know where you’re going, AI can’t help you get there.
I’ve found that “negative” prompts, where you tell the AI tool what not to do, can be extraordinarily useful. For example, if you’re worried that a question might subtly push respondents toward a certain answer, you can tell the model, “Avoid wording that suggests a positive (or negative) experience.”
Make It a Conversation
As I said earlier, surveys and LLM chats are both basically conversations. But, unlike surveys, AI tools allow for an ongoing back-and-forth. Take advantage of this. If ChatGPT or Claude aren’t giving you quite what you want, explain to the model what’s going wrong.
Now, admittedly, some days it simply feels like the AI models are off their game or even obstinate. But often, a pointed follow-up or redirect will get the conversation back on track.
Use AI Where It Helps
As you work with AI tools, you’ll learn more about where they shine and where they fall flat. Once, during a busy week, I tried using an LLM to generate an article abstract for me. When I sent it to a colleague, he said that it was clearly written by a robot. So, I don’t use AI tools to write my abstracts. But if I need to trim 50 words from an overlong draft, I go straight to ChatGPT.
Related Article: Top 10 AI Marketing Analytics Tools
Have AI Grade Its Own Work
You know that feeling you get when you’ve been working on a project for so long that you can’t really see it anymore? Things can get even blurrier when you’ve been working on something with AI, and you can’t even remember which ideas were originally yours and which were suggested by the LLM.
In this case, you can simply have the AI model evaluate its own work, perhaps using a gold-standard survey as a quality benchmark. But fair warning: I’ve found that AI tools sometimes give work produced by AI high marks, even when the quality isn’t where it needs to be. You can use the platform to provide initial feedback, but you’re going to have to make the final call.
As large language models become more sophisticated, the way we design and field surveys is evolving rapidly. Tools like ChatGPT and Claude offer new opportunities to streamline workflows, test assumptions and elevate the quality of research. But to realize their full potential, researchers must develop a new layer of skill, one that includes, but goes beyond, traditional methods. Techniques like prompt engineering are quickly becoming essential for guiding AI outputs effectively, and staying ahead will require ongoing education and adaptation.
Survey design has always been both art and science. In this new era, it also demands fluency in how to collaborate with intelligent tools.
Summary of Key Lessons: Designing Better Surveys With Generative AI
Editor's note: This table outlines the common pitfalls and best practices when using LLMs like ChatGPT or Claude to support customer survey design.
Mistake or Challenge | Recommended Practice | Why It Matters |
---|---|---|
Starting with poor or vague input | Provide high-quality reference materials (e.g., past surveys, trusted sources) | Stronger inputs help LLMs mimic voice, tone and accuracy, producing more useful drafts |
Using unfocused prompts | Be specific—narrow the topic, audience and format (e.g., "trust in primary care doctors") | Vague prompts lead to “hallucinations” or generic, low-value output |
Failing to set clear expectations | Define both positive and negative prompt instructions, including what to avoid | Helps steer the AI toward unbiased, targeted results and avoids skewed responses |
Treating AI as a one-shot solution | Engage in iterative conversation—follow up, clarify and course-correct as needed | LLMs improve with feedback; back-and-forth helps refine quality |
Misusing AI for tasks it doesn’t handle well | Identify where AI is strongest (e.g., trimming text, drafting ideas) and where it struggles (e.g., writing nuanced abstracts) | Knowing tool limitations ensures quality and saves time |
Losing track of AI vs. human contributions | Have the AI grade its output against gold-standard benchmarks—but review manually | AI can overrate its own work; human judgment remains essential |
Relying on outdated skills alone | Develop prompt engineering skills and continue learning as AI evolves | Survey design now requires fluency in how to collaborate with intelligent tools |
Source: MIT News