Universities offer quick-hit studies in AI, machine learning
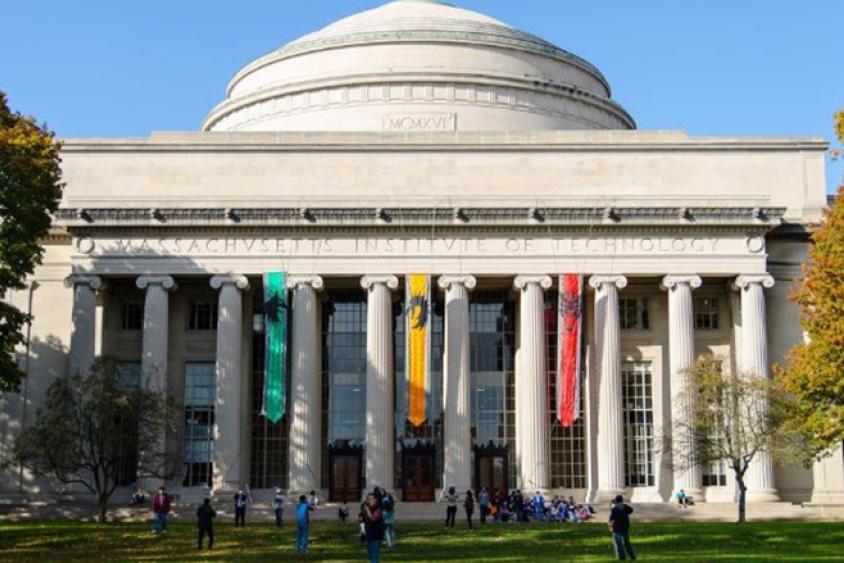
Organizations are desperate to use advanced technologies to help them gain competitive advantage, get closer to customers and boost the bottom line. In response, colleges and universities are rapidly expanding their course offerings in such topics as artificial intelligence, advanced analytics and machine learning.
A prime example is the Massachusetts Institute of Technology, which has announced seven new courses added to its 2018 Short Programs, covering emerging fields and technologies including AI, machine learning, automation, computational design and more.
To get a better idea of what is being taught in these programs, Information Management spoke with Lily Fu, director of open enrollment programs at MIT, and Justin Solomon, assistant professor of electrical engineering and computer science at the Computer Science and Artificial Intelligence Laboratory (CSAIL) at MIT.
Information Management: What are the “short programs,” and how do these new classes relate to what the Institute was already offering in these subject areas?
Lily Fu: Short Programs are offered through MIT Professional Education. The program includes more than 50 courses and three professional certificates designed to keep businesses and their employees up to date on cutting-edge practices and techniques.
This year, we added more than a half-dozen new courses to address the growing market demand for Artificial Intelligence and Machine Learning skills. With the skills shortage becoming increasingly apparent and competition for talent -- training in AI and ML has become a priority for companies in every industry around the globe.
Leaders need to understand how machine learning is evolving, what AI is, what Natural Language Processing is what Robotics is and so forth. The really important thing is to understand how the technology connects to their work.
Many people in industry struggle to keep up with the extremely fast pace of technological innovation. Our goal is to separate the wheat from the chaff, helping people to understand the big themes, how they relate to their own products and services, what they need to learn, and to acquire the broad set of skills needed to lead teams better and make better decisions.
All of the courses, which are taught by full-time MIT faculty and experts in the area, are constantly being updated with MIT’s latest research. Faculty work hard to get the latest practical angle for industries, with a focus on hands-on application. Participants don't just go to lectures, but they get to practice the techniques and apply them immediately to their job.
IM: Professors Justin Solomon and Suvrit Sra will be teaching a new class on Modeling and Optimization for Machine Learning. Who is that course targeted to, and what will students be learning in the class?
Justin Solomon: This course is for a relatively broad audience: engineers, programmers, data scientists, researchers, consultants, analysts, etc. – but it’s not for anyone. You definitely need some computer science or math background to benefit from the course.
Those who come will learn mathematical modeling, which is the skill of reducing a messy engineering or computational problem to a mathematical form that can be solved by using standard algorithms.
Since an important part of optimizing machine learning is developing the basic approach -- modeling, optimization and algorithm selection are critical to integrating deep learning and other machine learning into the business environment. By recognizing common patterns, participants will develop an intuition for which problems are solvable using standard modeling techniques and gain the knowledge and skills to solve them.
Our objective is to help students to identify the right tool from the modern modeling and optimization toolbox. We will be looking at tools for convex optimization, machine learning, social networks, online learning, adversarial networks, generative models, and emerging applications.
Practical takeaways will include experimental exposure to open source machine learning and optimization tools, modeling, and experience in how to recognize and identify which tools are relevant to a given problem.
IM: What is new with machine learning technology in 2018, and where to you see the technology headed in the next two or three years?
Justin Solomon: We’ve seen a broad range of AI applications over the past few years – from self-driving cars to healthcare. However, to date, much of the focus has been on algorithms -- when in reality, speed and power consumption is equally important.
A navigation system, for example, must process data in real time and can quickly drain smartphone batteries. These challenges are creating limitations in how we deploy machine learning and AI in the real world.
Consequently, in the next few years, I believe we will witness the emergence of specialized hardware systems that are custom designed to meet the speed and energy consumption requirements of AI and machine learning. We’ll start to see more interplay between hardware and algorithm designers with the goal of creating a new overall architectural approach.
IM: What do you believe are the business benefits of an organization embracing machine learning, and what are some examples of how it can boost efficiency, productivity or profitability?
Lily Fu: The possibilities and applications are endless. Many mundane tasks will be automated to free up time for breakthrough kinds of activities that didn’t exist before. AI will help identify new ways to improve operations and efficiency. It will allow organizations to save costs and move into new lines of business.
Change is already underway in a number of fields, including retail and manufacturing. You not only can make complex products that couldn’t be made before, but each product that comes from the assembly line can be different from the next to meet the need of any given customer. Whether it is custom-made shoes and apparel or on-demand automotive parts. And these are products that are being manufactured on-demand by robotic systems.
IM: Do you view machine learning as a competitive differentiator for an organization, and if so, what should the organization do to be most successful with it?
Lily Fu: It’s hard to overstate the importance of machine learning and AI in our digital economy. But for businesses, the challenge isn’t just developing or deploying the right technology – it will be ensuring they have the right talent in place.
The skills gap is real. Technical skills remain limited and are still evolving. As a result, the gap between ambition and execution is still pretty large at most companies. If organizations want to make good on the promise of AI, they will need to develop and/or hire people who understand AI and machine learning at a more advanced level. In my view, success will rely not only on innovation, but also long-term training and workforce development to establish that pipeline.
Source: Digital Insurance