MIT expert on the future of AI: A key hurdle stands on the path of innovation
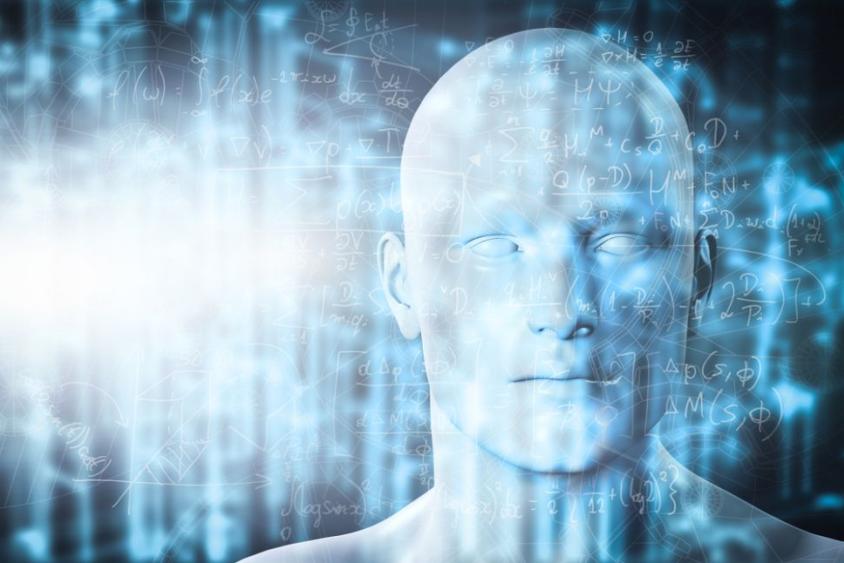
Advances in deep learning will help propel the future of AI.
Speed and energy consumption. These are two of the greatest challenges people face when deploying deep learning solutions. Fact is, while highly accurate, deep learning algorithms are complex and require more computation than other approaches. The analysis of massive data sets can lead to high power and heat dissipation in data centers which limits processing speeds; always-on applications can quickly drain power and memory resources in portable devices, such as smartphones and wearables. That limits real-world applications, particularly on mobile and handheld devices. One of the greatest limitations of progress in deep learning is the amount of computation available.
That’s why Vivienne Sze, Associate Professor of Electrical Engineering and Computer Science at MIT, decided to launch a new professional education course titled, Designing Efficient Deep Learning Systems. The two-day class, which runs March 28-29, at the Samsung Campus in Mountain View, California, will explore all the latest breakthroughs related to efficient algorithms and hardware that optimize power, memory and data processing resources in deep learning systems.
In the following interview, Professor Sze shares her thoughts designing efficient systems and how it is a crucial step toward wider deployment of AI applications.
There is a growing number of courses being offered today that focus on machine learning and artificial intelligence, what makes your course different?
Most courses fall into one of two categories: algorithmic design or computer hardware design. My course looks at how to make these two fields work together. How can you design algorithms that map well onto hardware so they can run faster and more efficiently? And how can you design hardware to better support the algorithms?
I felt there was a strong need for a course that teaches the interaction between both these disciplines. In other words, helping people understand how to jointly design both the algorithms and the hardware so they are better suited for deep learning. This knowledge will help address the challenges that one is faced with when deploying solutions in the real world.
What’s missing in today’s deep learning system design? Where can we make improvements?
There are various things we can do to optimize the algorithms, such as changing the number of layers, or shapes of filters to reduce memory access. But you can also design hardware that focuses on minimizing data movement, which dominates energy consumption. Make the network smaller, but equally accurate. Participants of this course will walk away with a better understanding of all the key considerations and options, as well as the trade-offs that come with each. The goal is to have your cake and eat it too: simultaneously achieve high accuracy while also meeting energy and speed requirements.
In my view, there are five key factors you need to consider in order to have a usable and feasible deep learning system. These metrics must be considered when evaluating or designing deep learning systems, and they are outlined below. Again, the goal is to have an efficient trade-off between all these different metrics.
- Cost – one primary cost is the cost of the chip itself. Chips that are larger with a higher number of cores and more memory are more expensive.
- Accuracy - you want to ensure the system you are using will produce sufficient accuracy based on the data set you are using for each particular task.
- Programmability - make sure the systems are flexible enough to support multiple applications and different weights.
- Throughput/Latency – For real-time applications, you want your hardware to operate at a reasonable speed; for instance, with video you want to reach a frame rate of 30 frames per second. For interactive applications such as navigation, you also need to consider the minimum reaction time.
- Energy/power – embedded devices have limited battery capacity, and in the data center you don’t want to generate too much heat.
Why are people so excited about deep learning? And how do you think the future of AI will pan out in the future?
Deep learning is a very popular approach for artificial intelligence, and it is becoming much more ubiquitous in our daily lives. It is also evolving very rapidly, and I believe it is poised to change the path of technology over the next decade because it allows computers to accurately extract meaningful information from the massive volume of data being generated every day, including videos, images, speech and more. AI is already everywhere from Google image recognition to self-driving cars to language translation. I’ve never seen so much excitement and promise. But as innovation continues, people must understand there are also limitations that, if not addressed, could prevent us from realizing the full potential of deep learning.
If we want to unleash the power of AI on the world, we must first build more efficient systems that support these complex neural networks on IoT enabled mobile devices, such as smartphones, wearables, drones and smart cars – where you have limited resources in terms of energy, storage and computation power. Advances in deep learning will surely enable many useful and exciting applications – such as computers that can see for the blind or drive autonomous vehicles. I truly believe these innovations will have a positive impact on society in years to come.
Vivienne Sze, Associate Professor of Electrical Engineering and Computer Science at MIT.
Source: IT Pro