Four Key Barriers to the Widespread Adoption of AI
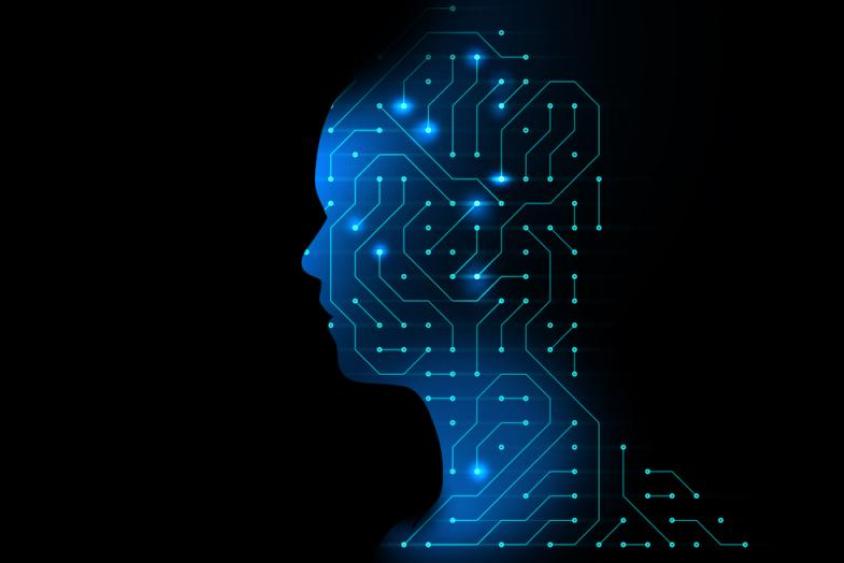
There is a lot of buzz about the promise of artificial intelligence (AI) and machine learning—from self-driving cars to predicting heart attacks, AI is spreading like wildfire across industries, triggering a massive investment in talent as businesses rush to adopt and/or deploy the technology.
But while there is growing excitement around what new applications AI will enable next, the reality is many issues and limitations still exist. Following are several key challenges that must be overcome before we can realize AI’s truly amazing applications.
Missing and disparate data
The availability of greater volumes and sources of data is enabling capabilities in AI and machine learning. There is a seemingly endless torrent of data being collected, but it remains incredibly hard to integrate that data. Information is often spread across multiple applications in various formats, including video, photos—making it hard to wrangle it all together while ensuring the quality of information.
On the flip side, certain industries, such as healthcare, still suffer from a lack of data. Experts know success with AI relies on having enough quality data to build models that provide meaningful learning and results. Just like the human brain needs information to develop, AI can’t develop properly without a certain quantity (and quality) of data. Organizations looking to adopt AI often find it is not only difficult to aggregate data across multiple medical record systems, but they are also limited by missing and incomplete data. For example, it is difficult to predict outcomes of rare diseases because there are very few patient data sets to pull from. It is also difficult to make a reliable decision about disease progression when a patient’s medical records aren’t linked together across providers and specialists. Luckily, new methods and techniques are helping professionals overcome these challenges.
Complexity challenges
Turns out, the more machines “know”— the less we understand them. Deep learning methods used for AI involve creating complex, hierarchical representations from simple building blocks to solve high-level problems. The network learns something simple at the initial level in the hierarchy, then sends the information to the next level where the information is combined into something more complex. The process continues, with each level building from the input it received from the previous level.
But the deeper the layers get, the harder the optimization algorithms become. Eventually, they become so hard that data scientist can’t explain how they work. At a certain point, the algorithms used for deep neural networks somehow, miraculously, produce accurate results—but scientists and engineers don’t fully understand how. AI enables machines to make predictions, but it is hard to get a computer to explain how it arrived at a conclusion. It not only raises trust issues, but also creates potential legal and liability issues. There is much to explore and consider in this regard before AI can move forward full force.
Time and energy limitations
Much of the focus around AI has been on developing better algorithms. But what many fail to consider is that not every system is designed to effectively deploy AI solutions. A crucial step toward enabling wider deployment is redesigning hardware to support AI, particularly for embedded applications like mobile technologies and drones. It’s an issue that is too often overlooked. There are also storage challenges. For AI innovation to continue, we must blaze new paths in the terrain of computer hardware design.
The skills gap
Among the biggest barriers to deploying AI tools is the talent shortage. Many businesses are struggling to secure employees with the combination of skills and knowledge necessary to unleash the full potential of AI. Consequently, more organizations are devoting more time and resources to training and development to establish an AI talent pool from within. The focus here needs to be on providing professionals with real-world experience that is both cutting-edge and practical and can be immediately put to use.
The race for AI is now underway. The technology is rapidly reshaping the boundaries of what we think of as possible in way that is both awesome and exciting, but there are several obstacles standing in the way of widespread adoption. Many of these challenges can—and will—be overcome, but it will take better data, better algorithms and better hardware. We can achieve all of those things if we commit to sharing knowledge and information that enables. This will enable better trained talent who can usher us into a new golden age of AI.
Lily Fu is the Director of Open Enrollment Programs at MIT Professional Education.
Source: RD Magazine