Revolutionizing Materials Science: An Interview with Markus Buehler on the Impact of AI
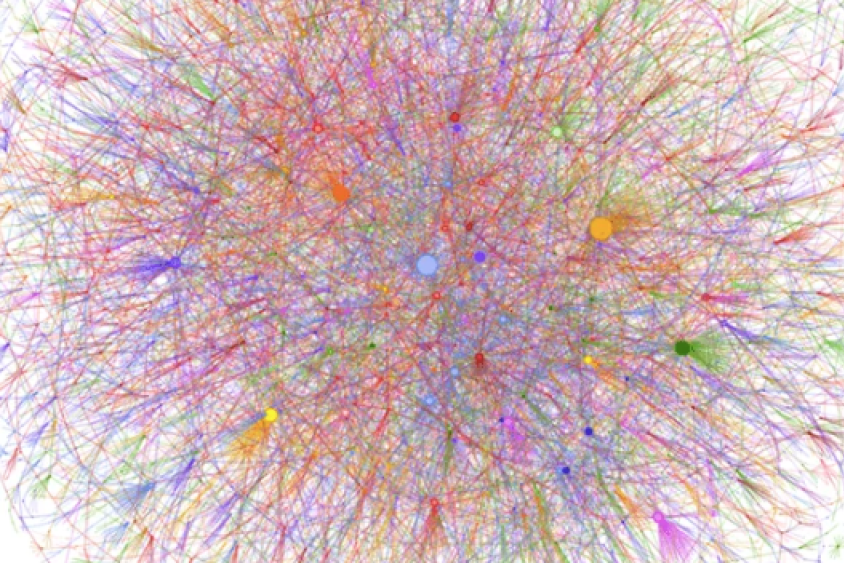
How has AI fundamentally altered the approach to materials design?
AI, primarily through generative AI models, has dramatically changed our approach by accelerating the design process significantly. These models can predict material properties from extensive datasets, enabling rapid prototyping and evaluation that used to take years. We can now iterate designs quickly, focusing on the most promising materials early in the development phase, enhancing both efficiency and creativity in materials science. This is a huge leap forward because it reduces the time and cost associated with traditional materials development, allowing for more experimentation and innovation.
What are some of today's most impressive state-of-the-art tools and applications in AI-driven materials science?
One notable application is using deep learning models to infer the internal properties of materials from surface data. This technology is groundbreaking, particularly for industries like aerospace and biomedical, where non-destructive testing is crucial. These models can predict internal flaws or stresses by analyzing external properties without physically altering the material. This capability is essential for maintaining the integrity of critical structures and devices, making materials safer and more reliable while saving time and resources. Other recent advances are in multimodal AI, where such models can design materials and understand and generate multiple input and output types, such as text, images, chemical formulas, microstructural designs, and much more.
Integrating AI into materials science must present some challenges. Can you discuss these?
The integration of AI brings challenges, particularly regarding the interpretability of AI models. Engineers and scientists must understand how predictions are made, which is not always clear with complex AI models. This opacity can make it difficult to trust and rely on AI outputs, especially in fields where material failure could have dire consequences. Addressing this challenge involves creating more transparent AI systems that offer insights into their decision-making processes, building trust and facilitating their adoption in sensitive and critical applications. One such way by which we are addressing this is by using several interacting AIs that challenge each other’s predictions, and that introduce physics and math into the solution process, to teach AIs how to ‘think’and reason with proper scientific grounding.
With AI opening new frontiers, what unique opportunities are now available in materials science?
AI enables the design of materials with customized properties tailored to specific applications, a significant advancement. For instance, we can now design lighter, more durable, or thermally resistant materials based on the requirements of specific applications like renewable energy technologies or electronic devices. Moreover, AI’s capability to explore and suggest novel material combinations across vast ranges of scales offers the potential to discover materials with properties that we have not even considered before, paving the way for innovations that could transform entire industries.
Looking to the future, what role do you see AI playing in materials science?
AI is set to play a transformative role in discovering new materials and optimizing manufacturing processes, especially when we deploy multiple AIs that interact, referred to as multi-agent AI. By leveraging multi-agent AI, such a system no longer relies solely on training data but can generate its own data by writing code, running new simulations, and developing new feedback about how these materials work. In the development phase, we can achieve higher precision and efficiency, reducing waste and enhancing productivity. Additionally, as we refine AI technologies, they will enable the molecular-level design of materials, offering precise control over their properties. This could lead to breakthroughs in numerous areas, such as energy storage, where enhanced materials could lead to more efficient batteries and other storage solutions.
Could you tell us more about MIT's Machine Learning for Materials Informatics course? What topics are covered?
The course focuses on teaching machine learning fundamentals and applications in materials science. It covers various topics, from the basics of machine learning techniques to advanced applications in materials informatics, such as multimodal LLMs. We explore how these technologies can predict material properties, design new materials, and even reverse engineer existing ones. The course includes hands-on labs where participants apply these techniques to real-world problems, using state-of-the-art software and tools, and how engineers can fine-tune their own LLMs and other generative tools. It is designed to empower professionals with the skills needed to lead the innovation of materials design using AI.
What specific skills or knowledge will participants gain from this course at MIT?
Participants will learn to apply machine learning models to complex materials science challenges across all scales. They will gain expertise in managing large datasets, using AI to predict material behaviors, and implementing inverse design techniques. The course also emphasizes the synergy between traditional materials science and modern computational methods, encouraging a holistic approach that integrates both fields. This will enable participants to push the boundaries of materials science, driving innovation in their respective areas. I pay particular attention to giving participants a deep understanding of how modern generative AI models work so that they can be fully informed about their potentials, risks and how to best use them for materials science applications.
How do you believe educational programs like this one impact the broader materials science community?
Educational programs like this are crucial for advancing the field. They help bridge the gap between traditional materials science methods and modern computational approaches, equipping professionals with the necessary tools to innovate effectively. By fostering a better understanding of how AI can be integrated into materials science, these programs enable the community to tackle new challenges more creatively and efficiently. They also help in cultivating a generation of materials scientists who are well-versed in both the theoretical and practical aspects of AI applications in materials design.
Lastly, what excites you most about the convergence of AI and materials science?
The most exciting aspect is the potential for discovery, including new knowledge and solutions. AI opens up possibilities that go beyond human intuition and conventional experimentation. It allows us to explore vast combinations of materials and properties at an unprecedented scale. I am particularly excited about the potential for AI to uncover new materials that could solve critical global challenges, like developing sustainable materials, dealing with the challenges of a changing climate, or new energy sources. The ability of AI to accelerate these discoveries enhances our scientific understanding but also has the potential to make a significant impact on our society and environment, and the innovation ecosystem.
Source: AZO Materials